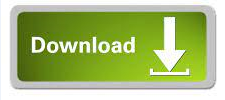
Xingxuan Zhang, Renzhe Xu, Han Yu, Hao Zou, Peng Cui. NICO++: Towards better bechmarks for Out-of-Distribution Generalization. Xingxuan Zhang, Yue He, Renzhe Xu, Han Yu, Zheyan Shen, Peng Cui. Who should be Given Incentives? Counterfactual Optimal Treatment Regimes Learning for Recommendation. Haoxuan Li, Chunyuan Zheng, Peng Wu, Kun Kuang, Yue Liu, Peng Cui. Propensity Matters: Measuring and Enhancing Balancing for Recommendation. Haoxuan Li, Yanghao Xiao, Chunyuan Zheng, Peng Cui, Peng Wu. Provably Invariant Learning without Domain Information. Xiaoyu Tan, Yong Lin, Shengyu Zhu, Chao Qu, Xihe Qiu, Yinghui Xu, Peng Cui, Yuan Qi. Renzhe Xu, Haotian Wang, Xingxuan Zhang, Bo Li, Peng Cui.Ĭompeting for Shareable Arms in Multi-Player Multi-Armed Bandits. He is now a Distinguished Member of ACM and CCF, and a Senior Member of IEEE. He is PC co-chair of CIKM2019 and MMM2020, SPC or area chair of ICML, KDD, He received ACM China Rising Star Award in 2015, and CCF-IEEE CS Young Scientist Award in 2018. His recent research won the IEEE Multimedia Best Department Paper Award, SIGKDD 2016 Best Paper Finalist, ICDM 2015 Best Student Paper Award, SIGKDD 2014 Best Paper Finalist, IEEE ICME 2014 Best Paper Award, ACM MM12 Grand Challenge Multimodal Award, and MMM13 Best Paper Award. He has published more than 100 papers in prestigious conferences and journals in data mining and multimedia.
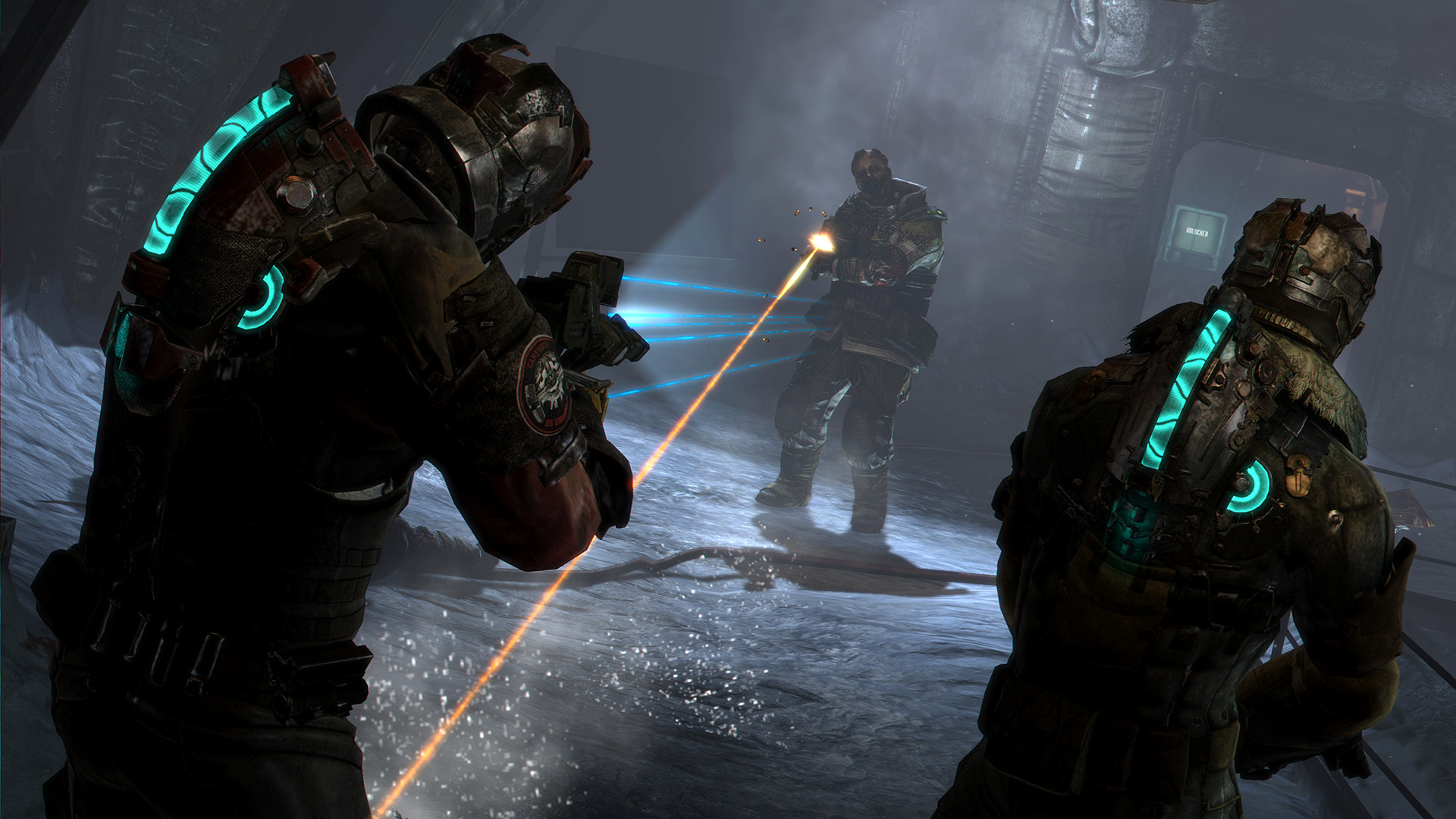
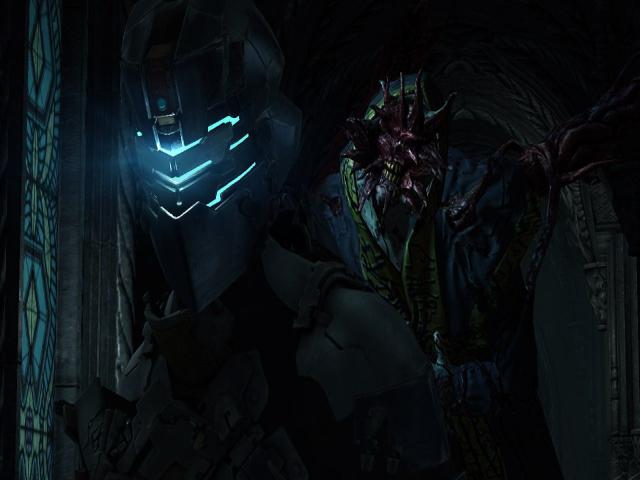
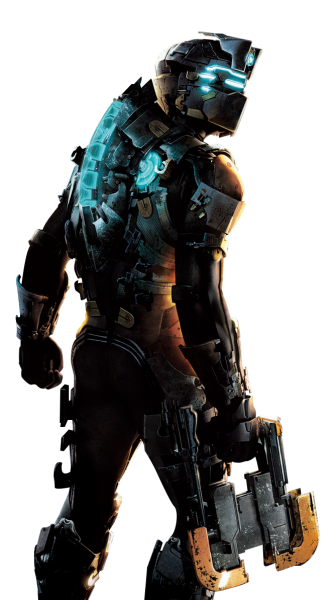
His research interests include causally-regularized machine learning, network representation learning, and social dynamics modeling. He got his PhD degree from Tsinghua University in 2010.

Peng Cui is an Associate Professor with tenure in Tsinghua University. We published a survey paper on out-of-distribution generalization here, accompanied with a website to maintain the paper list for OOD generalization. Together with Susan Athey, we published a perspective paper on stable learning in Nature Machine Intelligence. We have kicked off the NICO Challenge, a contest for OOD image recognition. Attention: We have several openings for Postdoctoral Fellowships on causal learning and related topics.
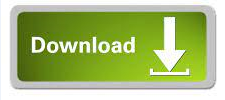